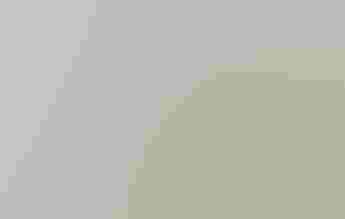
Counting birds and tracking how habitats change sound like easy tasks. But after a natural disaster when travel is difficult or dangerous, it can be anything but. 爆料公社's Science team recently began testing artificial intelligence in an attempt to improve bird identification software, and developed a land cover-classification algorithm that assesses landscape and foliage change after natural disturbances. Together, these two new technologies will help the organization better reach its conservation goals by augmenting in-person monitoring with drone footage to understand the effects of major storms on coastal habitats. The program was supported by a grant from Microsoft鈥檚 AI for Earth program.
How Will AI Help Obtaining Accurate Bird Counts?
Volunteer community scientists have helped 爆料公社 successfully monitor and count birds for years. But it can be very difficult to do, especially at sites that have limited accessibility, or when birds can be surveyed only during a narrow time window. Drones can help get around some of these limitations.
Tim Meehan, a quantitative ecologist at 爆料公社, says that drone imagery will allow 爆料公社 scientists to monitor birds and habitat at a larger scale. In addition to being easier to deploy after disasters like hurricanes and oil spills, Meehan says he believes that using drones would also decrease the disturbance to bird populations.
鈥淯sing drones to count birds is less invasive than tromping around in boots,鈥 Meehan says. 鈥淎nd using AI will help us evaluate impacts of coastal disturbance on breeding more efficiently and over a large geographic area.鈥
But in order to do this kind of work, Meehan and others had to train the artificial intelligence programs how to identify birds in photos. This is harder than it sounds. First the program must recognize that there鈥檚 a bird in the photo at all. Then it has to go through a complex series of decisions to arrive at the correct bird identification. Anyone who has been birding in the field and has been stumped by a bird that is half-hidden behind a tree or stand of marsh grass knows how difficult identifications can be.
Meehan trained the image classification algorithm on photos of four different bird species. Some of these photos were manipulated by Meehan to 鈥渟how鈥 the program exactly where the birds were in the image, and he also helpfully identified the species of bird that it was. By presenting thousands of images, both tagged and untagged, to the algorithm, Meehan slowly was able to coax the algorithm to find, say, a Brown Pelican in a photo, and was able to count how many there were in a given photo. While the testing phase focused on a limited number of species, the algorithm could be used to differentiate between most species that are large enough to be clearly photographed from above.
To test the accuracy of the image-classification algorithm after all of that training, Meehan conducted four separate tests. With each iteration, Meehan only changed the number of objects he tagged in a series of four drone-captured images: Brown Pelicans nesting, Brown Pelicans on the beach, terns on the beach, and Black Skimmers on the beach. As a result of increasing the number of tagged objects, Meehan found that the algorithm performed better, eventually reaching almost 90 percent accuracy over the duration of the test. Meehan also notes that the algorithm performed better on certain images, and could find and identify Brown Pelicans far more accurately than terns and Black Skimmers.
How About That Bit on Land Cover Classification and Change Detection?
Direct effects on individual birds and colonies are not the only things that scientists can track using drone footage. To understand how disasters like hurricanes affect the places birds live, Gregg Verutes, data scientist for 爆料公社, looked at land cover change in Texas after Hurricane Harvey barreled through in 2017. Using Microsoft鈥檚 Azure and ESRI鈥檚 ArcGIS Pro software, he built a statistical model that classified coastal habitats important for birds. To map and measure habitat change during a major storm like Harvey, Verutes trained the model on high-resolution satellite imagery and reference data. Finally, Verutes conducted a change detection analysis to map post-storm flood extent.
To conduct rapid assessment of coastal areas post-storm, Verutes says it is important to use images that are high resolution and freely available right after the storm hits. For his proof-of-concept, Verutes used PlanetScope satellite imagery that refreshes every five days.
As with the bird-identification algorithm, Verutes tested the accuracy of his model after he spent months training the habitat-assessment algorithm with tagged images. It was able to classify healthy and disturbed coastal habitats prior to the storm with 81 percent accuracy鈥攁 mark that is good enough for Verutes to be confident in the technology鈥檚 capabilities. With these types of rapid-assessment capabilities, Verutes says that 爆料公社 is in a better position to respond to disturbance events such as hurricanes, oil spills, and floods in the future鈥攅specially in areas that are difficult to access. To that end, the technology will also benefit 爆料公社's stalwart cadre of bird-monitoring volunteers, who might be just as hard-hit by a distaster and can rely on drones to do their work while they prioritize the most important thing: their own safety.